Machine Learning Malware Detection: Transforming the Future of Cybersecurity
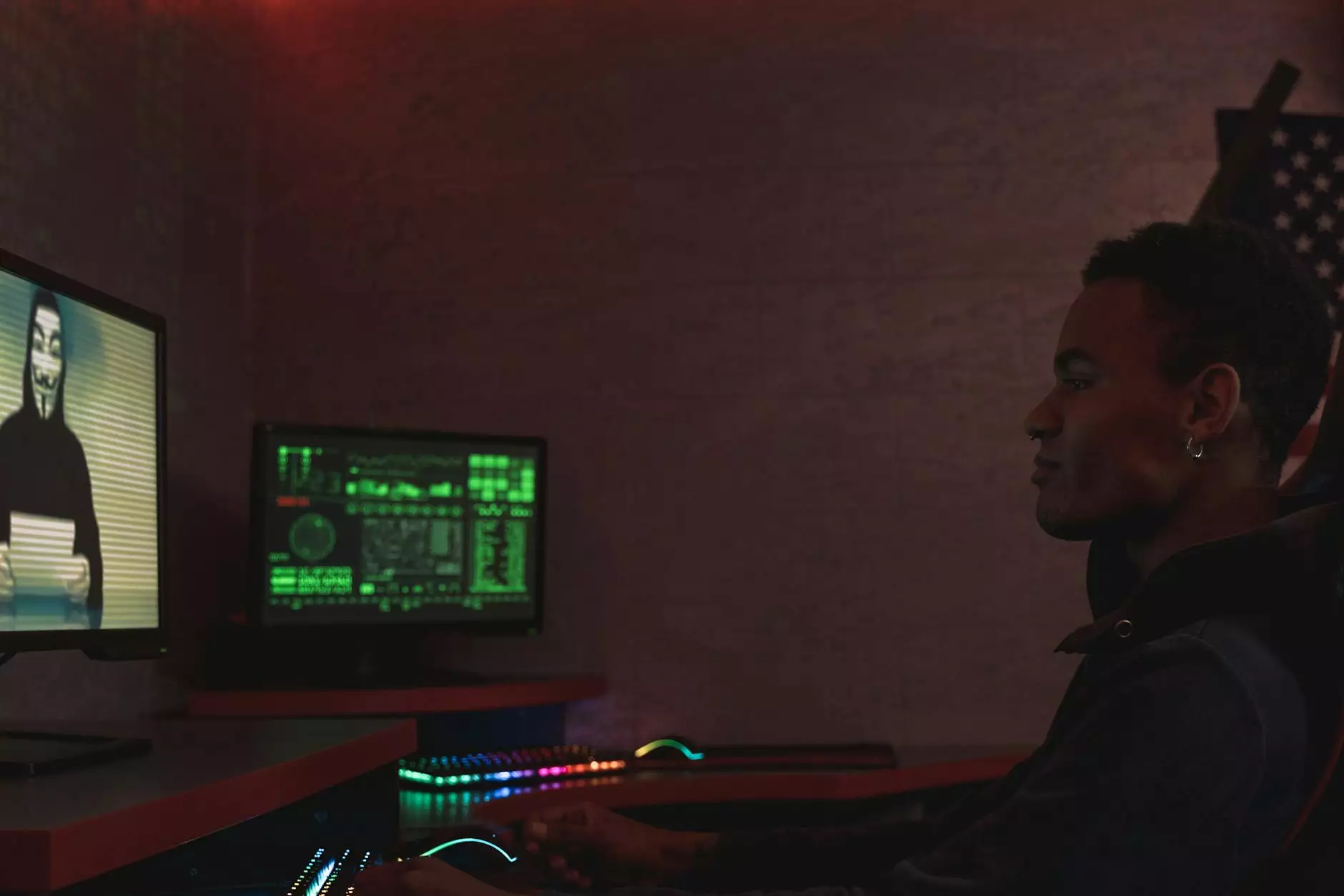
In today's digital age, *cybersecurity* has become a cornerstone of business operations. As threats evolve and become more sophisticated, organizations are in a continual race against time to safeguard their data and systems. At Spambrella, we harness the power of machine learning malware detection to provide unparalleled protection against cyber threats.
Understanding Malware and Its Impact on Businesses
Malware, or malicious software, is designed to disrupt, damage, or gain unauthorized access to computer systems. The implications of malware can be devastating, leading to data breaches, financial loss, and reputational damage. It is critical for businesses to understand the different types of malware:
- Viruses: Programs that can replicate themselves and spread to other systems.
- Worms: Stand-alone malware that replicates without human interaction.
- Trojan Horses: Malicious programs disguised as legitimate software.
- Ransomware: Malware that encrypts a user's data and demands payment for decryption.
- Spyware: Software that secretly gathers user information.
The complexity and variety of these threats require advanced techniques for detection and prevention, making machine learning an essential tool in cybersecurity.
Introducing Machine Learning in Malware Detection
Machine learning (ML) is a subset of artificial intelligence that enables systems to learn from data and improve their accuracy over time without being explicitly programmed. In the realm of cybersecurity, machine learning malware detection methods have proven to be a game-changer:
The Mechanism of Machine Learning Malware Detection
At its core, machine learning malware detection utilizes algorithms to analyze patterns in data collected from various sources, including files, system behavior, and network traffic. The process typically involves the following steps:
- Data Collection: Gathering extensive datasets that include both benign and malicious files.
- Feature Extraction: Identifying key characteristics of malware and non-malware samples, such as process behavior and code structure.
- Model Training: Using labeled datasets to train machine learning models to recognize patterns associated with malware. Common algorithms include decision trees, random forests, and neural networks.
- Detection and Classification: Once trained, the model can analyze new files and classify them as benign or malicious based on learned patterns.
- Continuous Learning: The system adapts by learning from new data and evolving threats, ensuring that defenses are always up-to-date.
Benefits of Machine Learning Malware Detection
Implementing machine learning within your cybersecurity framework offers numerous advantages for businesses:
1. Enhanced Detection Rates
Machine learning systems can identify threats with remarkable accuracy, recognizing subtle patterns that traditional signature-based methods may overlook.
2. Reduced False Positives
By analyzing a more extensive dataset, machine learning algorithms can minimize the number of false positives, ensuring that legitimate applications are not mistakenly flagged as threats.
3. Real-Time Analysis
With the ability to process vast amounts of data rapidly, machine learning can provide real-time analytics and threat detection, allowing organizations to respond swiftly to potential threats.
4. Proactive Threat Hunting
ML-driven systems can identify emerging threats before they cause harm, allowing for proactive measures and significantly reducing the potential attack surface.
Challenges in Implementing Machine Learning Malware Detection
While the benefits of machine learning malware detection are significant, there are challenges that organizations must navigate:
1. Data Quality and Quantity
The effectiveness of machine learning models largely depends on the quality and size of the training data. Insufficient or biased data can lead to ineffective detection models.
2. Complexity of Implementation
Integrating machine learning into existing cybersecurity infrastructures requires specialized knowledge and skills, making it necessary for businesses to invest in expert personnel or training.
3. Adversarial Attacks
Malicious actors are also evolving, employing techniques designed to bypass machine learning defenses. As a result, continuous monitoring and updating of models are essential.
Spambrella’s Approach to Machine Learning Malware Detection
At Spambrella, we understand the importance of evolving with technology to protect our clients, which is why we have integrated machine learning into our cybersecurity offerings:
Custom Solutions Tailored for Your Business
Our team works closely with businesses to develop customized machine learning solutions that address their unique security needs. We focus on:
- Data Integration: Seamlessly integrating machine learning with existing systems.
- Continuous Learning: Ensuring our models are updated with the latest threat intelligence data.
- Comprehensive Reporting: Providing detailed analytics and actionable insights for informed decision-making.
Proven Track Record in Cybersecurity
With extensive experience in IT services and computer repair, as well as security systems, Spambrella has established itself as a trusted partner in safeguarding businesses from cyber threats.
The Future of Machine Learning Malware Detection
The realm of cybersecurity is constantly evolving, and as businesses become increasingly reliant on technology, the demand for advanced detection methods will continue to grow. The future of machine learning malware detection will likely see:
- Increased Collaboration: Service providers collaborating to share threat intelligence for enhanced machine learning training datasets.
- Greater Use of AI: AI-driven techniques being employed to automate responses and improve efficiency in threat management.
- Adaptive Learning Models: Models that evolve not just with new data but also with insights gained from past incidents.
Conclusion: Embrace Machine Learning for Robust Cybersecurity
In conclusion, the adoption of machine learning malware detection is not just a trend; it is a necessity for businesses aiming to secure their digital assets in an increasingly hostile cyber environment. At Spambrella, we are committed to delivering state-of-the-art cybersecurity solutions that empower businesses to thrive while staying secure. With our machine learning capabilities, we help you stay one step ahead of cybercriminals, ensuring your data remains protected, allowing you to focus on what you do best—growing your business.