Mastering Image Annotation for Machine Learning: A Comprehensive Guide
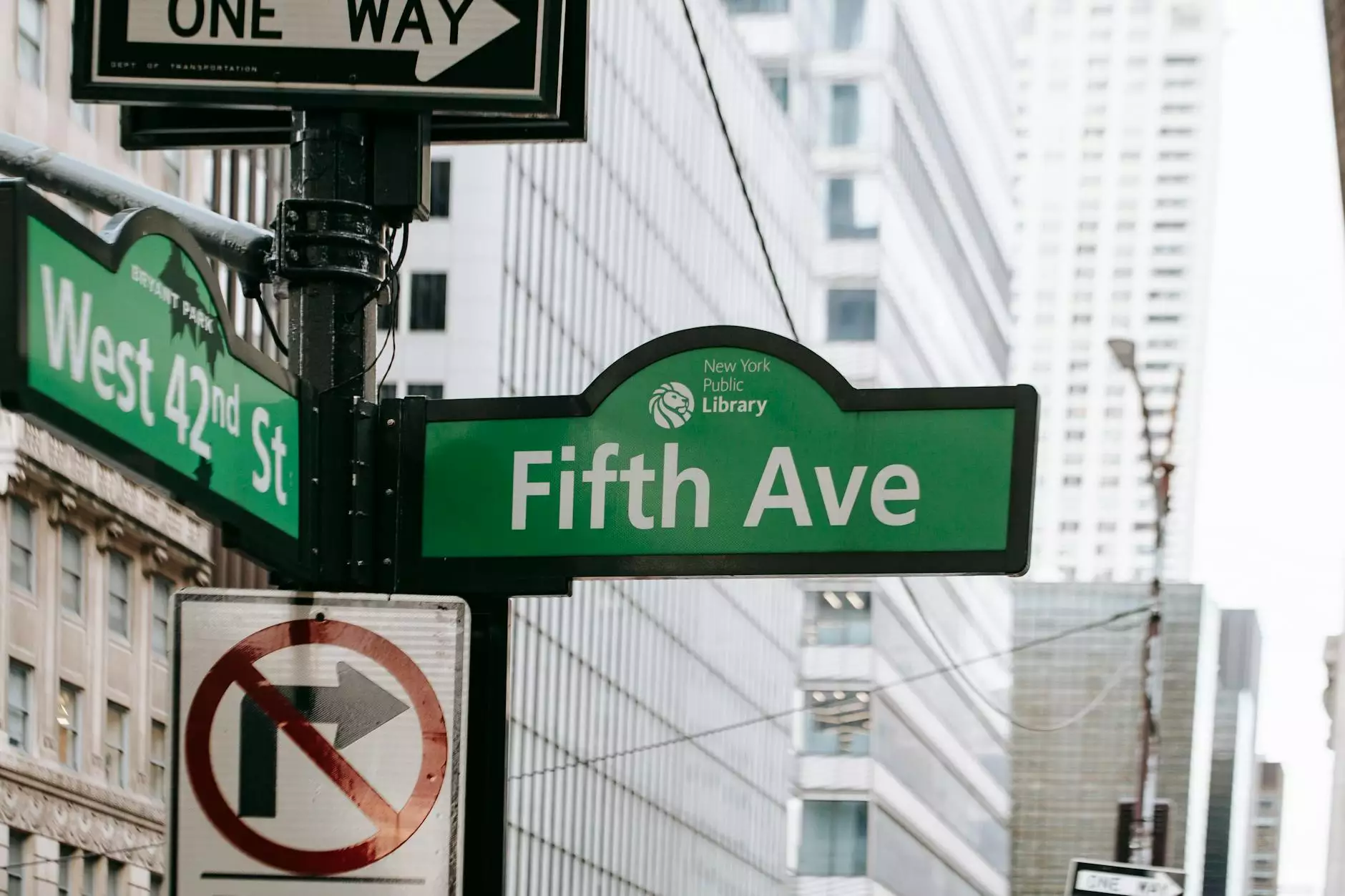
In the era of artificial intelligence (AI) and machine learning (ML), the term image annotation for machine learning has gained immense relevance. As we navigate through this digital age, it's essential to understand how properly annotating images can accelerate machine learning projects and ensure their success. This article delves into the intricacies of image annotation, its importance, the tools and platforms available, and the best practices for achieving optimal results.
Understanding Image Annotation
Image annotation refers to the process of labeling images with relevant tags and descriptions that help ML algorithms recognize and categorize visual data effectively. This critical step transforms raw data into a structured format that machines can understand, facilitating the training of AI models.
Why is Image Annotation Important?
- Enhances Model Accuracy: Properly annotated images provide the necessary context for machine learning models, leading to improved accuracy in predictions and classifications.
- Enables Supervised Learning: Supervised learning heavily depends on labeled datasets; image annotation is central to creating these datasets.
- Facilitates Real-World Application: High-quality annotations help AI systems perform better in real-world scenarios, making them more viable for businesses.
The Role of Data Annotation Tools and Platforms
The landscape of data annotation is continuously evolving, with numerous data annotation tools and platforms available to streamline the image annotation process. These tools not only automate parts of the annotation workload but also enhance collaboration among teams.
Key Features of Effective Data Annotation Tools
- User-Friendly Interface: An intuitive interface helps both beginners and experienced users manage their annotation tasks efficiently.
- Advanced Annotation Techniques: Tools that support various annotation types—bounding boxes, polygons, semantic segmentation—cater to diverse ML applications.
- Quality Control Mechanisms: Automatic validation checks and user feedback systems improve annotation accuracy and consistency.
- Integration Capabilities: The ability to integrate with existing ML frameworks and pipelines can significantly enhance workflow.
Popular Image Annotation Tools
Several tools stand out in the realm of image annotation, each offering unique features tailored to various ML projects:
- Labelbox: A collaborative tool that provides a seamless experience for labeling images and managing datasets.
- SuperAnnotate: Known for its advanced features such as AI-assisted annotation and comprehensive project management capabilities.
- CVAT (Computer Vision Annotation Tool): Open-source and customizable, ideal for teams looking for a flexible solution.
- Snorkel: Focused on programmatic labeling, Snorkel allows users to create labeling functions to automate the annotation process.
Best Practices in Image Annotation for Machine Learning
To maximize the effectiveness of your image annotation for machine learning projects, adhering to best practices is critical. Here are some vital strategies to consider:
1. Define Clear Guidelines
Establishing clear guidelines for annotators is essential to ensure consistent and high-quality annotations. Document specific rules regarding what constitutes an accurate label and provide examples.
2. Use Multiple Annotators
To mitigate bias and improve the accuracy of annotations, employing multiple annotators and conducting a review process can ensure that the labels are reliable and relevant.
3. Incorporate Quality Assurance Checks
Regular quality checks, such as double-checking a percentage of annotated images, can identify issues early on and maintain the integrity of the dataset.
4. Leverage Automation Where Possible
Utilizing AI tools to assist with initial annotations can speed up the process. However, always ensure human oversight is part of the workflow to catch any potential errors.
5. Continuous Training and Feedback
Offering continuous training and feedback for annotators can help improve their skills and ensure that they understand the evolving standards of the project.
Applications of Image Annotation in Machine Learning
The applications of image annotation for machine learning span various industries, enhancing capabilities in several domains:
1. Autonomous Vehicles
In the field of autonomous driving, image annotation is vital. Annotating images of road signs, pedestrians, and vehicles allows self-driving algorithms to recognize and react to their environment effectively.
2. Medical Imaging
Medical professionals utilize annotated medical images to train diagnostic tools. These annotations help in detecting anomalies like tumors or other abnormalities in radiological images.
3. Retail and E-commerce
Image annotation plays a significant role in improving customer experience in e-commerce platforms. By labeling products in images, AI can enhance search functionality and recommendations.
4. Wildlife Conservation
Conservationists use image annotation to label images captured in wildlife cameras. This process assists in monitoring animal populations and behaviors, contributing to conservation efforts.
Choosing the Right Data Annotation Platform
When selecting a data annotation platform for your projects, consider the following factors:
- Scalability: Ensure the platform can handle your projected workload, whether you're scaling up your project or working with large datasets.
- Support and Community: A robust user community and reliable support can help resolve issues quickly and improve your overall user experience.
- Cost-Effectiveness: Evaluate your budget and assess the pricing models to find a balance between features and affordability.
The Future of Image Annotation in Machine Learning
The future of image annotation for machine learning is bright, characterized by rapid advancements in technology and methodologies. As AI and ML evolve, so will the techniques and tools available for image annotation, enhancing their efficiency and effectiveness.
AI-Powered Annotation Tools
AI-powered annotation tools are set to revolutionize the field. These tools will leverage deep learning algorithms to automate much of the image labeling process, reducing costs and time while maintaining high accuracy levels.
Collaborative Annotation Platforms
With the rise of remote work and global teams, collaborative annotation platforms will allow multiple users to work simultaneously on annotation tasks, streamlining workflows and enhancing productivity.
Ethical Considerations
As AI continues to permeate various aspects of our lives, ethical considerations surrounding data privacy and bias in image annotation will gain prominence, necessitating transparent practices and guidelines.
Conclusion
In conclusion, mastering image annotation for machine learning is crucial for anyone involved in AI development. With the right tools, methodologies, and best practices in place, businesses can leverage the power of annotated data to build more accurate and reliable machine learning models. As the industry continues to innovate, staying abreast of trends and best practices will ensure that your projects remain competitive and effective. Embrace the future of machine learning with high-quality image annotation—your success depends on it. For further exploration and to discover top-notch annotation solutions, visit keylabs.ai.